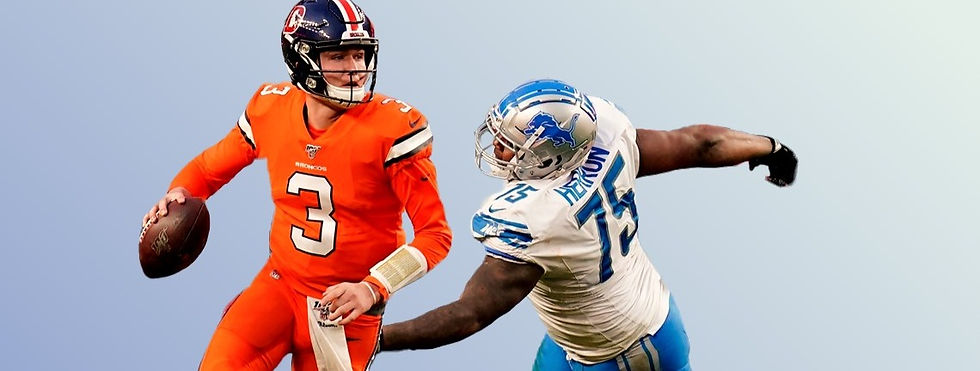
Drew Lock was the hardest quarterback to sack in the entire NFL last year. Harder to sack than athletic phenoms Patrick Mahomes, Lamar Jackson, and Josh Allen. Harder to sack than wily vets Tom Brady and Drew Brees. Of quarterbacks with at least 150 dropbacks, Lock's sack percentage outpaced the field by a full percentage point:

And on the rare occasions that he went down Lock was able to stay alive in the pocket longer than any other QB in the league.

Impressive stuff, especially for a rookie playing behind an offensive line that was struggling before he took over.
What should we make of this? We might conclude that Lock is a natural leader, and that his offensive line played harder for him than they did for Brandon Allen or Joe Flacco. Or we could conclude that Lock has an innate feel for navigating the pocket in the face of pressure. Or that his athletic ability helps him elude rushers. Or that he's an incredibly hard worker that reaped the benefits of intense film study and preparation. Or some mix of all those things.
But before reaching any of those conclusions, let's take a look at a pair of statistical and behavioral ideas.
Small Numbers
Of the 3,141 counties in the United States, kidney cancer is least common in counties that are:
Mostly rural
Sparsely populated
Traditionally republican
In the Midwest, the South, and the West
Why is that? Probably not because republican politics protect against cancer. But perhaps the fact that the counties are rural means that residents experience less pollution... Or have access to fresh foods without the sorts of chemicals and additives that might adversely affect the kidneys...
But let's also consider the U.S. counties in which kidney cancer is the most common. Those counties are:
Mostly rural
Sparsely populated
Traditionally republican
In the Midwest, the South, and the West
The exact same set of characteristics. Obviously, those reasonable-sounding explanations about pollution and diet don't explain what's going on. Rather, the explanation has to do with another of the features that the high-incidence and low-incidence counties have in common: they are sparsely populated. In other words, the sample size is small.
This is not a story about government policy or regional values/lifestyles. It's just a story about randomness and statistics. Specifically—and here's the key statistical idea—about the fact that small samples produce extreme results more often than larger samples.*
The Trap
People are hard-wired to look for causal explanations for things. (This is the key behavioral science idea.) When we read that the rate of kidney cancer is low in sparsely populated rural areas, it's almost reflexive for us to explain why that might be by zeroing in on some feature of the world.
This default mode of thinking contrasts with a statistical perspective in which each event is a randomly determined outcome selected from a huge number of alternatives. Think about what would happen if it were possible to run the same pass play over and over—the same offense against the same defense. Say we're able to run it 1,000,000 times. Of course, there would be lots of different outcomes. Overthrow, underthrow, interception, easy reception, diving catch, etc.
Imagine each of these 1,000,000 outcomes is written on a Post-it and dropped into a hat. The number of Post-its for, say, "interception" will depend on many things. The QB's accuracy, ability to read the defense both pre- and post-snap, his comfort going through progressions, the talent of the defensive backs and pass rushers, the talent of the wide receivers and offensive line, and on and on.
Now imagine reaching into the hat and selecting a Post-it. Whatever the proportions of the outcomes written on the slips of paper, the selection is random. This is how the statistical perspective understands events in real life as well: as outcomes that happened to occur from among many, many alternatives.
If you draw from the hat 900,000 times, you would have a pretty good sense of the distribution of outcomes. But if you only draw from the hat 90 times, it would be much easier to get a very weird set of Post-its that doesn't represent the full range of outcomes in the hat. Whatever you see when you look through those 90 Post-its would be best explained by the fact that you're only looking at a small set of the possible outcomes—not by the factors that determined the distribution of those outcomes in the first place.
This is a deeply unintuitive way of thinking about the world. But it highlights why small sample sizes are such a trap. At their core, they represent statistically random events, but because of the way our brains our wired we see them as causal—driven by observable features of the world around us.
Lock's Phenomenal (-ly Misleading) Sack Rate
Returning to Drew Lock's sack rates, it's easy to spot the trap at work. A Sports Illustrated article about Lock's rookie season contained the following sentence:
Although his sample size was significantly smaller than those QBs who were 16-game starters, his elusiveness and pocket feel was almost preternatural.
Despite identifying the small sample size, the author still attributes the sack rate to other, non-statistics stuff—in this case, Lock's "preternatural" "elusiveness and pocket feel". He can't help it! It's just so natural (for all of us!) to explain things by appealing to observable features of the world, like Lock's athleticism and mobility.
The article goes on to elaborate on Lock's magician-like escapability, but like the kidney cancer rates, the numbers that paint Lock as the hardest QB in the league to sack are all about statistics. Specifically, about small sample sizes yielding extreme results. They do not support a conclusion that Lock has exceptional feel for moving in the pocket, inspires elevated play from his line, or anything else of the sort.
Predicting Performance from Small Samples
And, of course, this is true not only of his sack rates, but of all the statistics he produced in his five game stint as a starter. They all have small sample sizes.
It's extremely difficult to predict a QB's performance based on a full season's worth of games, let alone just five. To consider just a few cases of the problems that small sample sizes present:
Marcus Mariota had a fantastic start to his career before crashing back down to earth and becoming a backup.
Baker Mayfield had a promising rookie season, but face-planted in year two.
Nick Foles was an unstoppable force in his first real year as a starter, but is clearly just a backup.
Speaking of Foles, Gardner Minshew II came out of the gates on fire as his replacement last year before cratering down the stretch.
And, closer to home, we are fans of an organization that considered signing Trevor Siemian to a longterm deal after his hot start, and that brought Case Keenum in to be "the guy" on the basis of a good fifteen game stretch.
On the flip side, Peyton Manning set the interception record his rookie year before going on to have a hall of fame career.
John Elway also had a terrible rookie season, completing only 47% of his passes before improving that figure by nearly ten percent as a sophomore.
Of course, each of those boom then bust QBs has some true talent level that has (or will) become apparent across a large sample size. Talent, teammates, scheme, coaching, health, etc. all influence outcomes, and the relative weight of each will become increasingly apparent the more games a guy plays. But the statistical perspective highlights that in a small sample, randomness drives outcomes. Results are more likely to be extreme—farther from a player's average—as the sample we consider gets smaller. And predicting future performance based on five (or even sixteen) games is accordingly fraught.
Expectations for Year Two and Beyond
All of this is not to suggest that Lock won't be the future at quarterback in Denver. Or that he didn't play well, or that he's likely to fail. There's a lot to like about the young signal caller, and I would bet on him to succeed in the long run (hiring Pat Shurmur to run the offense and adding a bunch of weapons really boosts my confidence). But at the end of the day, that's how we need to think of it: as a bet that has not yet been settled.
The point of this exercise is to accurately set our expectations. Lock's extraordinary sack-evading performance as a rookie does not mean he will be exceptional in that area going forward. It's far more likely that he is average in that area, and that his rookie numbers had more to do with statistical randomness than with his traits as a player or leader.
Lock still has questions to answer, and suggesting otherwise is misguided. He played well enough that we should absolutely continue to give him opportunities, and be optimistic about what the future might hold. While success is not a foregone conclusion, it's well within the range of outcomes. But let's expand that sample size a little bit before congratulating ourselves on finding a QB. Give ourselves another sixteen (hopefully nineteen 😉) more games before we throw that party.
*From Thinking Fast and Slow, by Daniel Kahneman
Comments